时 间:2023年02月28日(周二)15:00-16:30
地 点:李达三楼104室
主持人:复旦大学 管理学院 统计与数据科学系 蒋斐宇 青年副研究员
题 目:Universality of regularized regression estimators in high dimensions
报 告人:Qiyang Han
Assistant professor of Statistics
Rutgers University
个人简介:
Qiyang Han is an assistant professor of Statistics at Rutgers University. He received a Ph.D. in Statistics in 2018 from University of Washington under the supervision of Professor Jon A. Wellner, and a B.Sc in Mathematics in 2013 from Fudan University. His research expands broadly in mathematical statistics and high dimensional probability, with a particular focus on empirical process theory and its applications to nonparametric and high dimensional statistics. He is a recipient of the NSF CAREER award in 2022, and the Bernoulli Society New Researcher Award in 2023.
摘 要:
The Convex Gaussian Min-Max Theorem (CGMT) has emerged as a prominent theoretical tool for analyzing the precise stochastic behavior of various statistical estimators in the so-called high dimensional proportional regime, where the sample size and the signal dimension are of the same order. However, a well recognized limitation of the existing CGMT machinery rests in its stringent requirement on the exact Gaussianity of the design matrix, therefore rendering the obtained precise high dimensional asymptotics largely a specific Gaussian theory in various important statistical models.
This work provides a structural universality framework for a broad class of regularized regression estimators that is particularly compatible with the CGMT machinery. Here universality means that if a `structure' is satisfied by the regression estimator μ ̂_G for a standard Gaussian design G, then it will also be satisfied by μ ̂_A for a general non-Gaussian design A with independent entries. In particular, we show that with a good enough l_∞ bound for the regression estimator μ ̂_A, any `structural property' that can be detected via the CGMT for μ ̂_G also holds for μ ̂_A under a general design A with independent entries.
As a proof of concept, we demonstrate our new universality framework in three key examples of regularized regression estimators: the Ridge, Lasso and regularized robust regression estimators, where new universality properties of risk asymptotics and/or distributions of regression estimators and other related quantities are proved. As a major statistical implication of the Lasso universality results, we validate inference procedures using the degrees-of-freedom adjusted debiased Lasso under general design and error distributions.
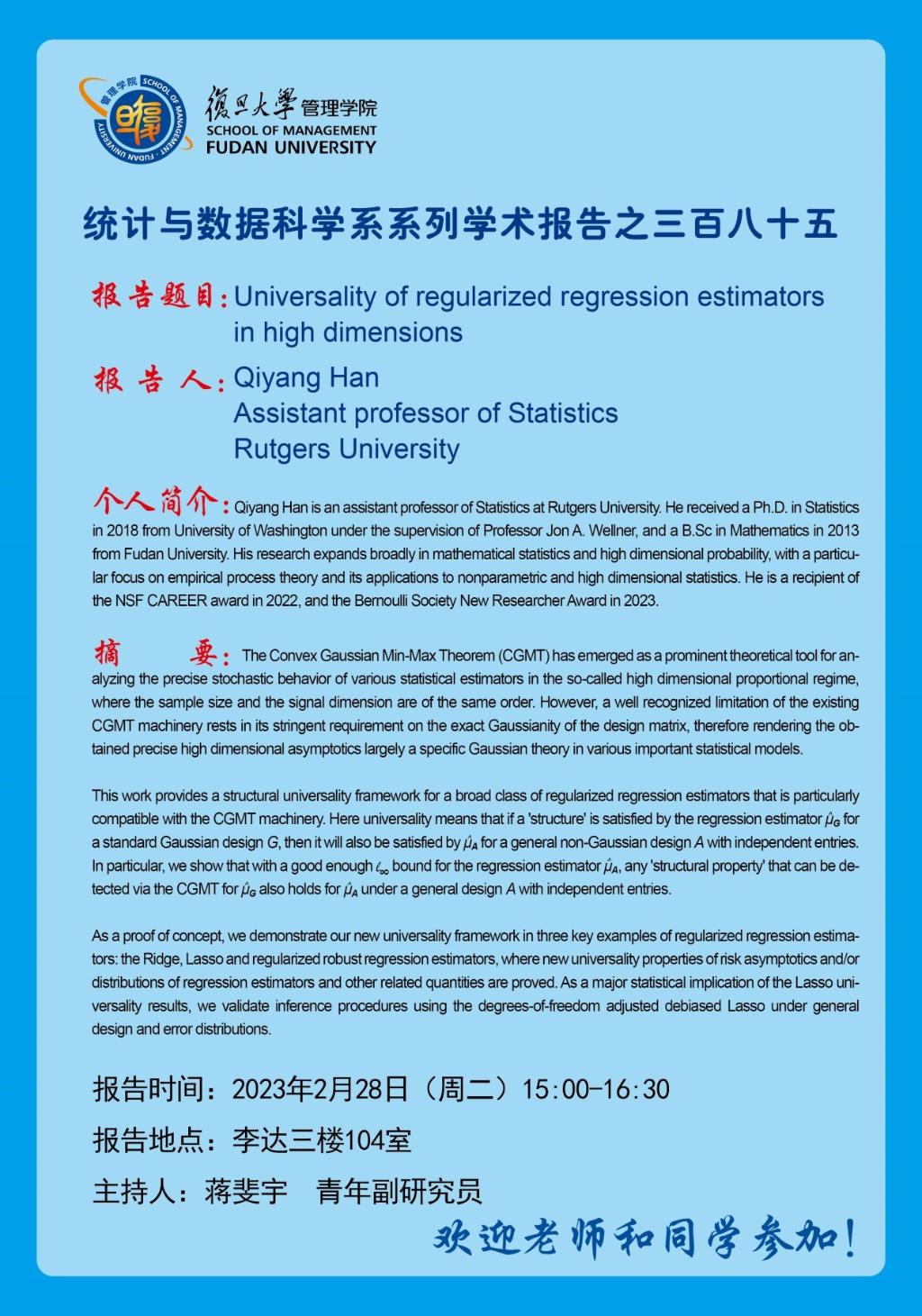
统计与数据科学系
2023-2-20